The fascinating world of robotics has made recent advancements that are not just about building smarter machines but about creating robots that can understand and interact with the world in ways we've only dreamed of. At the forefront of these developments is Google DeepMind's latest innovation, the Robotic Transformer 2, or RT-2 for short.
Google DeepMind Brief
DeepMind is an artificial intelligence research company founded in 2010 and acquired by Google in 2014. Here are some key facts about DeepMind:
- DeepMind was founded in London by researchers Demis Hassabis, Shane Legg and Mustafa Suleyman. Hassabis served as the company's CEO.
- The company specialized in developing AI algorithms that could learn and improve at tasks like a human. Their goal was to create artificial general intelligence (AGI).
- DeepMind's major breakthrough was creating AlphaGo in 2016 - an AI system that defeated the world champion in the complex game Go. This demonstrated sophisticated learning algorithms.
- Google acquired DeepMind in 2014 for over $500 million, attracted by its advances in deep learning and machine learning.
- Now an independent Alphabet company, DeepMind continues pioneering new AI capabilities in areas like protein folding, climate science and computer vision.
- DeepMind's notable AI products include the AlphaFold protein structure predictor, the MuZero game-playing system and WaveNet voice synthesis software.
- The company has faced criticism for its partnerships with healthcare organizations regarding data privacy and consent. But it continues pushing boundaries in safe AI development.
- Leading AI researchers like Demis Hassabis, David Silver, Koray Kavukcuoglu and many others continue to publish influential papers through DeepMind.
In summary, DeepMind is one of the leading AI research companies, known for breakthroughs like AlphaGo and for its acquisition by Google to boost its AI capabilities. It remains influential in guiding the development of safe and beneficial artificial intelligence.
RT2 is an experimental chatbot developed by Google that is based on their Responsible AI (RAI) research.
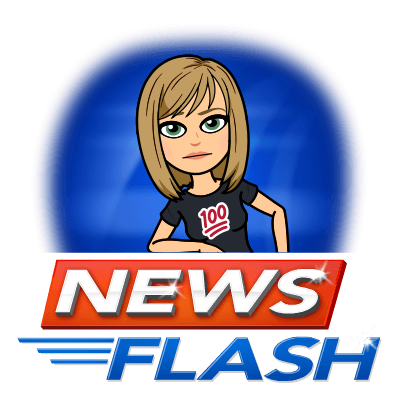
No, we are talking about the famous Italian TV Streaming service (RAI) but rather of a research in a multidisciplinary field that explores the ethical and societal implications of artificial intelligence (AI). It aims to ensure that AI systems are developed and used in a way that is fair, transparent, accountable, and beneficial to society.
RAI research encompasses a wide range of topics, including:
- Bias and fairness in AI: AI systems can perpetuate and amplify existing biases in society, leading to discriminatory outcomes for certain groups of people. RAI researchers are developing methods to identify and mitigate bias in AI systems.
- Transparency and explainability of AI: AI systems can be complex and opaque, making it difficult to understand how they make decisions. RAI researchers are developing methods to make AI systems more transparent and explainable so that people can understand why a particular decision was made.
- Accountability and responsibility for AI: As AI systems become more complex and autonomous, it becomes increasingly important to determine who is accountable for the decisions they make. RAI researchers are developing frameworks for assigning accountability for AI systems.
- Social and ethical impacts of AI: AI has the potential to have a profound impact on society, both positive and negative. RAI researchers are studying the potential social and ethical impacts of AI to inform the development and use of AI technologies.
For the average Joe of Robotics, that translates into a few projects that are currently using RAI:
- ProPublica's Machine Bias Calculator: This tool helps people to understand how AI systems may make decisions based on their race, gender, and other factors.
- Google's Fairness Testing Toolkit: This toolkit provides tools and resources for developers to test and mitigate bias in their AI systems.
- IBM's AI Explainability 360: This toolkit provides tools for developers to make their AI systems more transparent and explainable.
- The Algorithmic Justice League: This non-profit organization works to promote accountability and transparency in the development and use of AI systems.
- The Montreal Institute for Applied Ethics: This institute conducts research on the ethical and societal implications of AI.
These are just a few examples of the many RAI research projects that are underway. The field is constantly evolving, and new research is being published all the time.
Back to RT2
Imagine having a conversation with a friend or co-worker. You discuss topics like movies, sports, news, etc. The conversation flows naturally, with both of you understanding the context and responding appropriately.
Now imagine having that kind of smooth, human-like conversation with a computer program. That's essentially what Google's R2 chatbot aims to do.
R2 is an artificial intelligence system designed by Google researchers to have more natural conversations. When you chat with it, R2 tries to understand the context of the conversation and look up relevant information online to have coherent, in-depth discussions on different topics.
For example, if you start talking about basketball, R2 will know key facts about the NBA, famous players, team standings, and more. It will use that knowledge to have a conversation grounded in real information.
R2 represents a major advance in conversational AI. Previous chatbots were limited to short, simple exchanges. But R2 can carry longer, more robust conversations thanks to its advanced design.
Under the hood, R2 uses a technology called transformers, which are powerful in processing language. It also retrieves information from the internet to enrich its responses, rather than just relying on its training data.
Fun Fact: The "T" in GPT stands for "Transformer". GPT, or Generative Pretrained Transformer, is a type of transformer model. It was developed by OpenAI, and it's designed to generate human-like text. Google invented the underlying technology of Transformers (thanks, Ashish Vaswani!) and made it available to the rest of the planet.
The goal is to create an AI assistant that can engage people in friendly, useful conversations on many topics. R2 is still experimental technology, but it gets us closer to that human-like chatbot experience many hope for in the future.
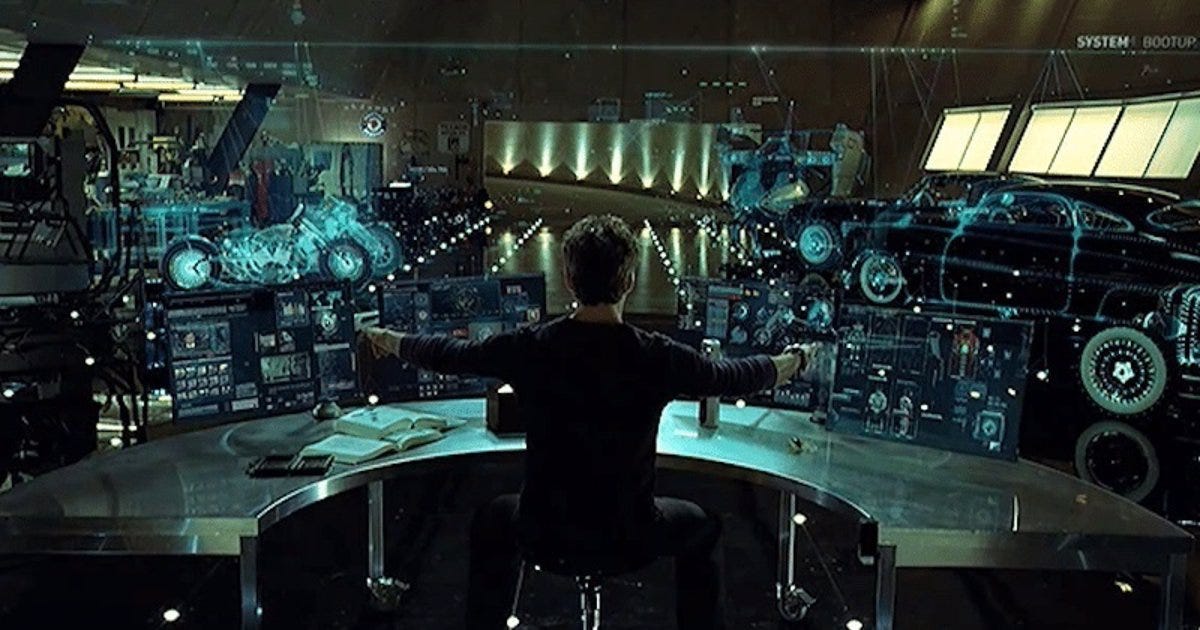
RT2 stands for "Retrieval-Tuned Transformer 2". It is the second generation of Google's chatbot that uses a retrieval mechanism and Transformer neural architecture.
- Unveiled in April 2022, RT2 is designed to have more human-like conversations by retrieving contextual information from the internet in real-time. This allows it to have more knowledge-intensive dialog.
- RT2 builds on Google's first-generation RETRO chatbot by significantly scaling up its parameters to 540 billion, making it one of the largest chatbot models.
- It was trained on dialogue data from Reddit to have more casual, open-ended conversations. RT2 can discuss movies, books, sports, politics and more.
- A key focus in developing RT2 was making it safer, more trustworthy and aligned with Google's AI Principles. It aims to avoid generating harmful, biased or untrue statements.
- RT2 is not currently available publicly. It is an internal research project at Google to advance conversational AI and address challenges like consistency, knowledge and safety.
What makes RT-2 so special?
In simple terms, it's a robot that can 'see', 'understand', and 'act'. Unlike traditional robots that just follow pre-set instructions, RT-2 can interpret what it sees, process language like we do, and then decide on the best course of action. It's like having a robot that can not only listen to our instructions but also understand the context and respond intelligently.
But why is this important? In our daily lives, we encounter countless situations that require understanding and adaptability. Imagine a robot in your home that can understand when you ask it to fetch a drink from the fridge or a robot in a factory that can adapt to new tasks on the fly. This is the kind of future RT-2 is ushering in.
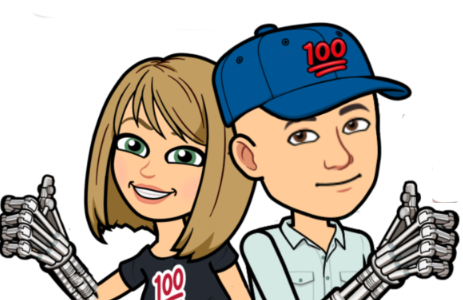
DeepMind: Exploring The Innovator Behind RT-2
Before jumping into the cool bot talk, I want to take a step back and put Google's DeepMind company in the spotlight. They are not just any out of the cookie-cutter company, they are a beacon of innovation in the world of artificial intelligence. Before being acquired by Google, they were founded in London, England and had been making headlines with their groundbreaking developments in AI.
You may remember seeing or hearing about the ALPHGO competition back in 2016? Well, this is the company that was the innovation behind the brains of it.
Besides that highly coveted match, they have made plenty of innovations. For those who want a little more information on that, below is more achievements.
DeepMind's 5 Biggest Achievements at A Glance
1. AlphaFold
One of DeepMind’s biggest breakthroughs in the last decade was an AI program called AlphaFold, which launched on 22 July 2021. AlphaFold uses AI to process the amino acid sequence of proteins and predicts the shape of proteins by generating a 3D model.
Before the release of AlphaFold, scientists only knew the 3D structures for just 17% of proteins in the body. Now after the launch of AlphaFold, scientists have access to over 200 million protein predictions, and 98.5% of 3D structures for human proteins can be predicted.
2. AlphaGo
Another research project that gathered significant international interest is AlphaGo. AlphaGo is an AI-driven program that uses machine learning and deep neural networks to play the board game Go. AlphaGo analyzes past games and board configurations to predict the next move to take when playing Go.
Unlike other AI-playing Go programs, which used search trees to test all possible moves and positions, AlphaGo was given a description of the Go board as input and then trained to play against itself thousands of times to improve its decision-making capabilities.
3. WaveNet
WaveNet, released in 2016, is another one of DeepMind’s core creations. It is a generative model for raw audio that is trained on a large volume of speech samples and has the ability to generate natural-sounding speech based on text or audio input.
Instead of cutting and recompiling voice recordings like other text-to-speech systems, WaveNet instead used a convolutional neural network trained on images, videos, and sounds to learn and emulate the structure of human language.
This meant that it could compose waveforms from scratch and generate speech that mimics the sound of a human voice. Now, WaveNext is used in a range of popular applications, including Google Assistant, Google Search, and Google Translate.
4. Google Bard
In the generative AI era, one of Deepmind’s most important contributions has been its work on the Google Bard chatbot, which was released in partnership with Google AI in March 2023.
Bard is built on the Pathways Language Model 2 (PaLM), a language model trained on publicly-available data, including web pages, source code, and other data, and enables users to process users’ natural language queries and responses in natural language.
5. RT-2
Just months after working with Google AI to release Bard, DeepMind proceeded to release RT-2 in July 2023, the first vision-language action (VLA) robotics transformer model. RT-2 processes text and images taken from across the web and uses them to output robotic actions.
RT-2 can be used to control robotics equipment, teaching robots how to do basic tasks, such as identifying a piece of trash and throwing it away. It also has the ability to respond to user commands with reasoning in the form of object categories or high-level descriptions.
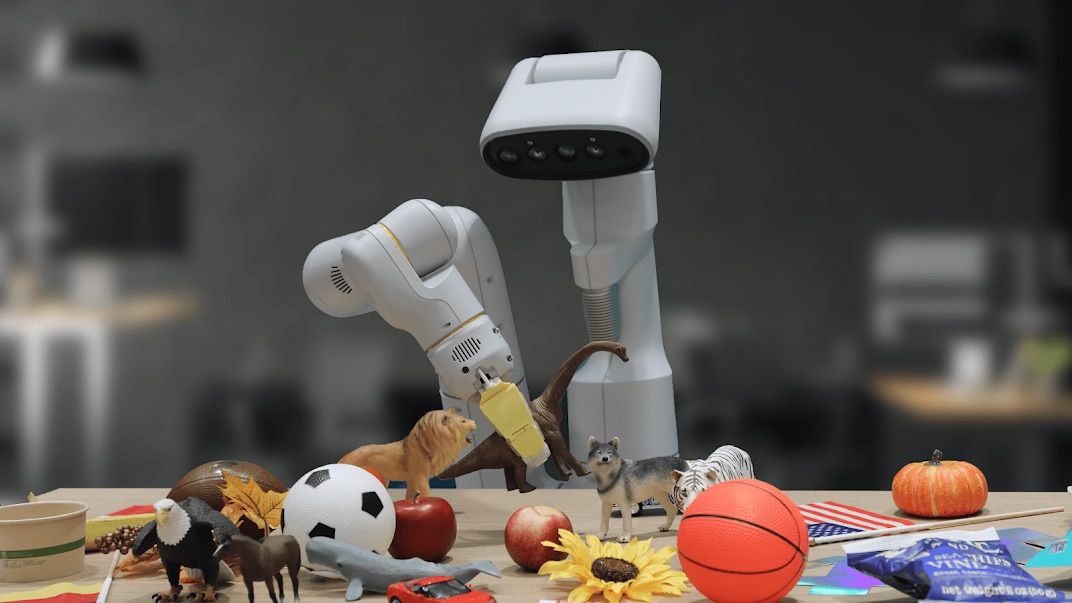
Let's Dive In: Technical Overview
I know the robot is awesome-looking and cute and all that good stuff to post a selfie with. But this robot is way more than that, let's explore the technical intricacies of RT-2. Its key features, and the immense potential it holds and what this fascinating world, where the lines between robotics, AI, and human-like understanding continue to blur, paving the way for a future filled with intelligent, adaptive robotic companions. The technical prowess of RT-2 stands out as a beacon of innovation. Understanding the mechanics behind this model is key to appreciating its groundbreaking nature.
Tech Specs
Main Feature | RT-2 Latest |
---|---|
Model Type | VLA Vision Language Action |
Learning Base | Integrates web & robotics data |
Generalized Capabilites | High Advanced Understanding & Adaptability |
Problem-Solving Abilities | Advanced chain of thought reasoning |
Adaptability | High- Can interpret & respond to new commands |
The Advent of RT-2
What sets RT-2 apart from other robots is its ability to integrate and learn from a vast array of web and robotics data. This isn't just about a robot learning from what it's been directly taught; it's about absorbing and applying a broader spectrum of knowledge. RT-2 embodies a more holistic approach to robotic learning and functionality.
Jumping into the deep side for the capabilities of RT-2, I couldn't help but be amazed by its potential. It's not just an improvement over RT-1—it's a completely new paradigm in robotic intelligence. RT-2's ability to understand and execute commands based on both visual cues and linguistic instructions opens up a world of possibilities.
Imagine a robot that can not only perform tasks it has been explicitly trained for but also apply reasoning to tackle tasks it has never encountered before. This level of adaptability and understanding in a robot was once a distant dream, but with RT-2, it's becoming a reality.
Building on a strong foundation
Just like any great robotic device it has to begin somewhere. That started with the introduction of RT-1 by Google DeepMind. This was a notable milestone model, trained on multi-task demonstrations, represented a significant shift in how robots could learn and adapt. It was impressive, observing how RT-1 could handle combinations of tasks and objects that it had encountered in its training data. But what truly intrigued me was the potential for what could come next.
A little clip about the first prototype RT-1
The Core Concept: VLA Model Explained
There are so many places to start with but at its heart, RT-2 is a Vision-Language-Action (VLA) model. This might sound technical, but the idea is straightforward yet profound. The model integrates visual understanding (Vision), linguistic comprehension (Language), and physical interaction (Action) into a cohesive framework. What does this mean for robotics? It's the convergence of seeing, understanding, and doing – all within one integrated system.
VLA Explained
The Vision-Language-Action (VLA) model is a concept in robotics and AI that integrates visual perception, language understanding, and physical actions. This model is especially relevant in the development of robots and AI systems that interact with humans and their environment in a more intuitive and effective manner. Here's a simple yet detailed explanation:
- Vision: In the VLA model, the 'Vision' component refers to the ability of a robot or AI system to perceive and understand its surroundings through visual inputs. This can be achieved through cameras or other visual sensors. The system processes these inputs to recognize objects, understand scenes, and navigate spaces.
- Language: The 'Language' aspect involves understanding and generating human language. This could mean comprehending spoken or written instructions, asking questions for clarification, or describing what it sees or plans to do. This is crucial for effective communication between humans and robots, making the interaction more natural and accessible for non-expert users.
- Action: Finally, the 'Action' part is about the robot or AI system performing physical tasks in the real world. This involves moving, manipulating objects, or changing its environment based on the information gathered from its vision and language understanding. The actions should be precise, safe, and aligned with the goals or commands given by human users.
In essence, the VLA model is about creating robots and AI systems that can see, understand, and interact with their environment in a human-like manner. This involves processing visual data, comprehending and using language effectively, and taking appropriate actions based on this understanding. This model is particularly significant in making robots more autonomous, adaptable, and helpful in everyday scenarios, such as in homes, workplaces, and public spaces. It's a step towards creating machines that can assist and collaborate with humans seamlessly and intelligently.
VLA explained
From Images to Actions: The Architecture Unveiled
RT-2's architecture, it's built upon Vision-Language Models (VLMs) that process images and output sequences of tokens, typically representing language. But RT-2 takes this a step further. It translates these tokens not just into words, but into actions. Think of it as teaching the robot a language where words are actions. This approach is revolutionary because it enables the robot to 'understand' commands in a more human-like manner and respond with physical actions.
This is more than just identifying an apple from an orange but a fresh apple to a bruised one. Think about one day, we can send RT-2 to the store to buy apples and it won't buy the bruised ones that had been dropped on the floor and put back on the table. Ahh now if I could only get my husband to do the same.
VLM Vision Language Model -The Quick Pro Quo
Vision-Language Models (VLMs) are a type of AI model that combines visual and linguistic information to understand and generate content. Here's a simplified explanation of their key components and functions:
- Vision Processing: VLMs analyze and interpret visual data, like images or videos. They use techniques from computer vision to identify objects, scenes, and activities within these visuals. This process is akin to how humans look at a picture and recognize various elements in it.
- Language Understanding: Alongside visual processing, VLMs are also equipped to understand language. This means they can read and interpret text, which could be descriptions, questions, or commands related to the visual content. This dual ability allows the model to bridge the gap between what is seen (the visual data) and what is said or written (the language data).
- Integration of Vision and Language: The core strength of VLMs lies in their ability to integrate visual and linguistic information. For instance, if shown a picture with a caption, a VLM can understand how the text relates to the image. Similarly, it can generate descriptions for images, answer questions based on visual content, or even create new images based on textual descriptions.
- Applications: VLMs have a wide range of applications. They can be used in image and video search, where the system understands queries in natural language and finds relevant visual content. They are also useful in automated content moderation, helping to identify and filter out inappropriate images based on understanding both the image and any associated text. Additionally, VLMs can assist in educational tools, providing visual aids and explanations to help users better understand complex concepts.
In summary, Vision-Language Models are advanced AI systems that understand and process both visual and textual information. They are valuable in various applications where the combination of visual and linguistic understanding is essential, making them increasingly important in a world where digital content is predominantly visual and textual.
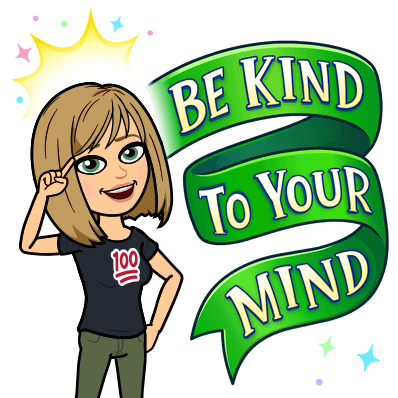
Chain-of-Thought Reasoning: A Game-Changer
One aspect of RT-2 that I find particularly fascinating is its chain-of-thought reasoning ability. This isn't just about following instructions; it's about reasoning through a problem. Let me repeat that once again...
IT'S ABOUT REASONING THROUGH A PROBLEM!!
For instance, RT-2 can decide which object could be used as an improvised hammer or what type of drink to offer a tired person. This level of reasoning in a robot is a giant leap towards more intuitive, human-like decision-making in AI.
I've been particularly impressed by how this model blurs the lines between digital processing and physical interaction. It's a testament to the incredible advancements we're witnessing in the field of robotics.
Up next, I'll delve into the key features and capabilities of RT-2, showcasing why it's not just another robot but a harbinger of a new era in robotics.
The future of AI and robotics is not just about technology; it's about reimagining the very essence of humanity. Stay curious for the next chapter. 🤖✨